AI + biobank
Introduce the human
element, sooner
The high failure rate in oncology drug development trials hints at a lack of drug-response data in a heterogeneous tumor population. This can impact even the most confident drug-discovery design. But what if you could introduce this valuable data well before clinical trials? With PEDAL you can. Our AI-driven platform is powered by a biobank of tumor samples to move molecules forward with a higher probability of success.
Improve the agility of drug discovery
Identifying which molecules will work on which cancer types can be like finding a needle in a haystack. PEDAL leverages incomplete data and efficiently picks the best new data needed to make drug response predictions. Our proprietary AI-driven platform, powered by our biobank, allows for optimized, high-confidence drug-response predictions within a large experimental space.
Increase the probability of success
PEDAL optimizes the drug development process by efficiently introducing tumor heterogeneity at pre-clinical stages. We base our AI models on something never before seen in this industry: tumor features derived from a biobank of over 150K+ tumor samples. With this platform, PEDAL's drug response predictions enable a more informed selection of drug/tumor combinations to increase the probability of success during development.
Repurpose drugs and improve portfolio diversity
With PEDAL, shelved and failed drug compounds can be repurposed through new evaluation in additional tumor types.
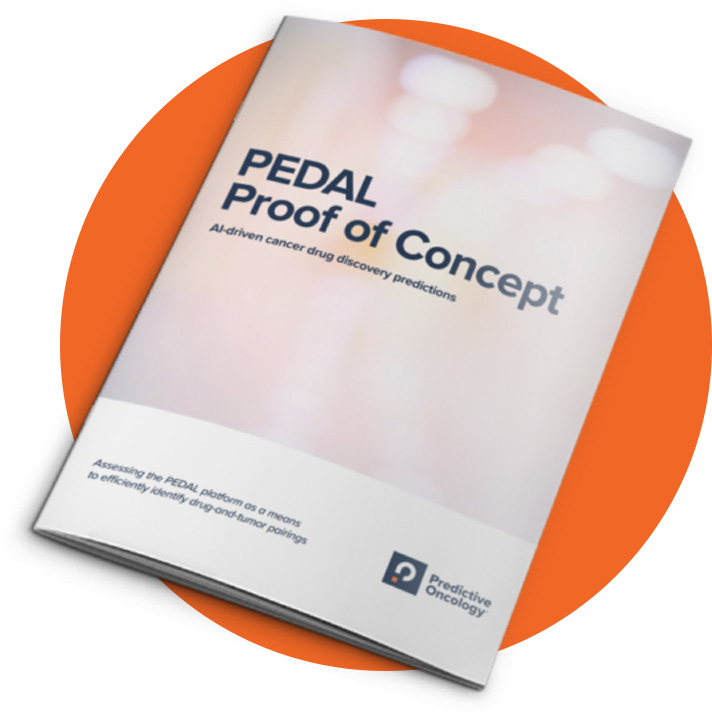
Predict tumor-drug pairing with 92% accuracy.
Learn how we assessed 175 existing, FDA-approved oncology drugs against 130 ovarian tumor samples with our Proof of Concept Study for PEDAL.
Artificial intelligence meets human diversity
While other AI companies purport to help speed and optimize the drug discovery process, PEDAL offers something never before seen in this industry: tumor features derived from a biobank of over 150,000 tumor samples from 137 cancer types.
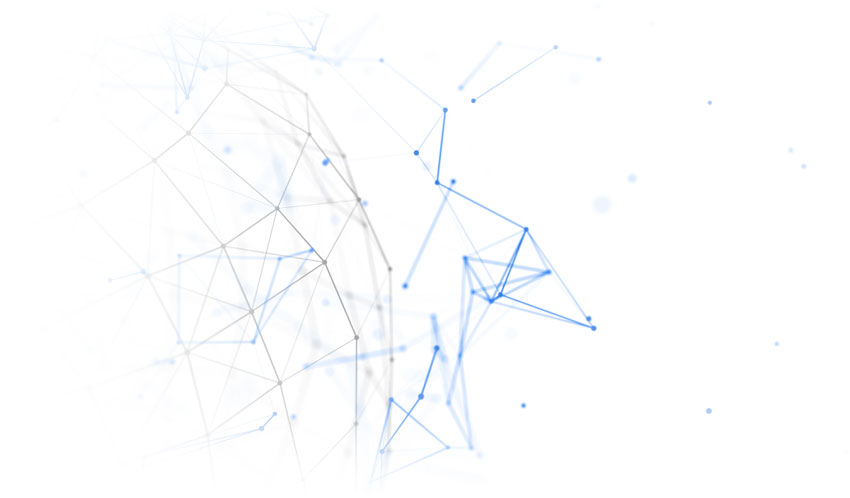
More about the AI engine that powers PEDAL.
Learn about our advanced AI machine-learning platform CORE™ (Computational Research Engine), developed by top researchers at Carnegie Mellon University and licensed exclusively to POAI. CORE takes an active machine learning approach that constructs models of all possible combinations of patient-specific drug response and uses these models to efficiently drive rounds of wet-lab drug-response testing in an iterative manner.
Start a PEDAL pilot program today
Complete the form and our PEDAL expert will be in touch to discuss customized solutions for your needs.
News & resources
“The way in which Predictive Oncology utilizes the power of predictive models offers the potential to optimize the utilization of resources, improve patient outcomes, and...
Life Sciences Knowledge Hub, recognized as the fastest-growing life sciences media platform, met with our Chief Executive Officer, Raymond F. Vennare, and Chief Business...
Our mission at Predictive Oncology is to change the landscape of oncology drug discovery and enable the development of more effective therapies for the treatment...